[pac_divi_table_of_contents included_headings="off|on|on|off|off|off" scroll_speed="8500ms" level_markers_3="none" title_container_bg_color="#004274" _builder_version="4.22.2" _module_preset="default" vertical_offset_tablet="0" horizontal_offset_tablet="0"...
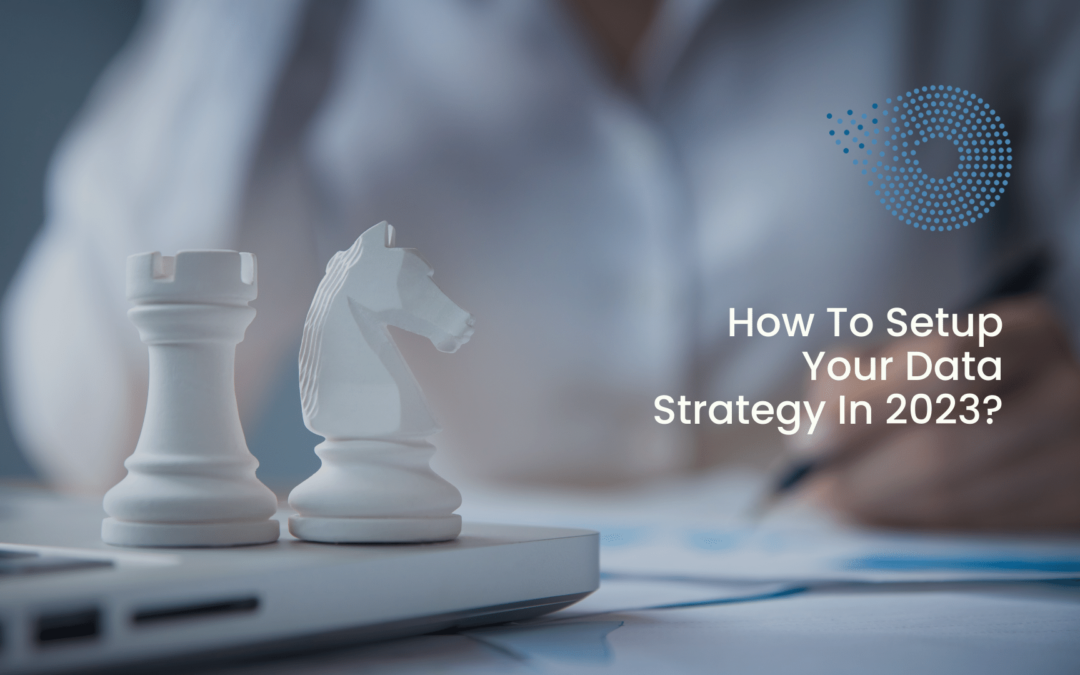
How To Setup Your Data Strategy In 2023?
Data is now recognized as one of the most significant assets for businesses in the current age of technology.
A recent IDC analysis estimates that by 2025, the amount of data generated globally will reach 175 zettabytes. Due to the data’s exponential increase, data strategy and its importance for corporate success get more attention.
Many major changes are influencing how businesses approach their data strategy. Increased use of cloud-based data platforms, a move toward real-time data analytics, a focus on data democratization, and more integration of AI and machine learning are some of these trends.
Why Is Organizational Alignment Important For Data Strategy?
The importance of organizational alignment is that it guarantees that everyone in the organization is working toward the same goals and utilizing data consistently and meaningfully. Alignment is crucial for an effective data strategy.
All organization stakeholders know the value of data and its role in accomplishing business goals when there is alignment around data strategy. Executives, managers, data analysts, and other employees work under this category.
Without alignment, several parts of an organization can be working toward competing objectives or might not be making the best use of data.
It might lead to inefficiencies, lost chances, and a general lack of progress toward corporate goals.
Different sets of data or processes by several departments or business units can create data walls, making it challenging to get a complete picture of an organization’s operations.
Also, alignment enables businesses to manage and reduce data strategy-related risk more effectively.
Shared knowledge of the risks related to data usage, such as privacy and data security concerns, exists when all stakeholders are on the same page.
It helps businesses to set up appropriate controls and governance frameworks to manage these risks efficiently.
Data Strategy Trends In 2023
#1 Shifting Workloads To The Cloud
Moving your systems to infrastructure hosted by the cloud could be enough to move workloads to the cloud.
It does address the issue of hardware upkeep and disaster recovery procedures, but you are not truly utilizing the full potential of the cloud.
Companies may benefit from moving workloads from a physical infrastructure to a cloud solution in various ways, including greater communication and flexibility, lower IT expenses, increased data security, and better scalability.
Businesses can process information more quickly and effectively, scale their infrastructure as necessary, easily operate complicated programs, back up important data, and use cloud data analytics tools for predictive insights.
#2 Removing Data Silos Throughout Departments
Within organizations, data silos are a significant barrier to data-driven decision making. These silos must be destroyed to encourage departmental collaboration.
Companies may create more accurate predictive models and obtain trustworthy insights from many sources by ensuring consistent data across the organization.
This strategy will enable teams across the organization to make the most of business data, feel confident in their choices, work together easily across teams, hit goals, and increase revenue for the company.
#3 Architecture for Data Mesh
It is a new development in data management that makes decentralized and more flexible data sharing possible.
It enables the construction of numerous data streams connected to various teams privately instead of centrally.
Data Mesh may appear to be an arrangement of segregated data at first glance, but this organizational structure allows various teams to analyze data to meet their fundamental needs.
And gives them the authority to maintain their data, which can subsequently be used as a source for analysis by other teams.
Also, when combined with Data Fabric and a data lake setup, data is managed for a centralized virtual system that stores the data, applies business logic, and provides data feeds to particular departments based on their requirements while remaining in source systems.
It makes high-quality data more widely accessible and reduces the time to value.
#4 Building a Modern Data Stack Infrastructure
Switching to a modern data stack requires examining your current tools and investing in new ones built for the cloud, and you can take advantage of how simple it is to add and remove computing, storage, and memory as needed.
Younger, agile companies have historically preferred the Modern Data Stack, whereas older corporate businesses prefer the singular accountability of a single vendor relationship.
But as the economy enters a recession, larger businesses begin to recognize the value a Modern Data Stack offers as they search for competitive advantage.
Organizations may create an architecture for real-time data analytics, machine learning, and effective data sharing by connecting multiple data sources.
Which Includes ERP systems, CRM databases, weblogs, IoT devices, and legacy systems.
But managing the growing volume of data from these various sources will be difficult for businesses, so it’s crucial to have the right technology in place from the beginning.
#5 Creating a Data Culture Across the Company
The Modern Data Stack’s main goal is to enable all employees to actively use data to improve their daily job and support data-driven decision making.
A culture of data can only be developed once this is realized and data is successfully shared across teams.
Leaders may assist in future-proofing the business, identifying effective development possibilities, uniting teams to achieve shared goals, and much more after this has been instilled into the organization and the value of data is understood and utilized across teams.
#6 Creating New Sources Of Income Through Data Monetization
Recent years have seen a rise in data monetization as businesses seek to profit from the huge amount of data they possess.
Teams with access to data from throughout the organization can benefit from marketing insights to support sales conversations, sales conversations to support customer service inquiries, and so on.
By analyzing this company-wide data set, leaders can find and create new products and services that answer unmet customer needs.
It presents an opportunity to carve out a niche in competitive marketplaces.
#7 Natural Language Processing (NLP)
It is an element of artificial intelligence that dedicates itself to recognizing and understanding human speech.
Since the early days of predictive text based on previous message writing to the continuously developing development of spam filters, email filtering, and pre-labeling, NLP has existed as a discipline.
With the introduction of self-teaching bots as opposed to the more traditional predetermined pathway bots, NLP is now becoming more popular in how people interact with data.
For business users who don’t want or need to learn how to utilize analytical tools, data exploration solutions like Thoughtspot and Tableau’s Ask Data integrate NLP into the workflow using a search bar-style interface to create analyses and get insight.
Although this requires a strong platform, data governance, and metadata management foundation, the advantages it can provide are considerable.
#8 Artificial Intelligence
For many companies, it is already standard practice to use AI and machine learning to find trends and patterns in data and produce insightful business information.
The idea of artificial intelligence, once thought to be a self-teaching generalist mind, has recently undergone a significant evolution in favor of smaller-scoped, more narrowly focused programs.
Businesses can begin to explore innovation in customer journeys, products, and services by allowing AI to handle routine tasks that can be time-consuming or where human error is most likely.
#9 Data Governance & Data Security
Data has a huge influence, but it also carries huge responsibilities. Businesses must ensure their data is protected as data breaches increase in frequency.
Your overall design must ensure that the appropriate individuals have access to the appropriate information at the appropriate time, not that everyone has constant access to all information.
Good data governance must be implemented to comply with GDPR and data security regulations to guarantee that data is managed correctly and is not misused.
Data governance is not a new trend in data, but because of how important it is, it will always be among our top 10.
How To Organize Your Organization Around A Data Strategy
A planned and active method must be used to align a company around a data strategy. The following actions can be made to make sure that all organization stakeholders support the data strategy:
- Establish clear goals and objectives for the data strategy
- Develop a data governance framework
- Define roles and responsibilities
- Communicate the data strategy
- Provide training and support management.
- Keep a record.
These actions can guarantee that stakeholders support the data strategy and work toward set objectives.
It could encourage data management that is more effective, efficient, and innovative, all of which could increase the organization’s overall success.
Conclusion
Organizations that can successfully match their data strategy with their overarching business goals will have an important advantage in the constantly developing field of data strategy, which offers significant prospects.
The landscape of data strategy in 2023 will be shaped by the trends we’ve covered in this blog, such as the growing importance of data governance, the value of data ethics, and the adoption of emerging technologies like AI and machine learning.
Recent Post
AI Personalization Excellence: Enhancing Customer Engagement in 2024
Accounts Payable vs. Accounts Receivable: Differences Explained with Example
[pac_divi_table_of_contents included_headings="off|on|on|off|off|off" scroll_speed="8500ms" level_markers_3="none" title_container_bg_color="#004274" _builder_version="4.22.2" _module_preset="default" vertical_offset_tablet="0" horizontal_offset_tablet="0"...
Custom AI Solutions – Tailoring AI to Your Unique Needs in 2024
[pac_divi_table_of_contents included_headings="off|on|on|off|off|off" scroll_speed="8500ms" level_markers_3="none" title_container_bg_color="#004274" _builder_version="4.22.2" _module_preset="default" vertical_offset_tablet="0" horizontal_offset_tablet="0"...